\(\newcommand{\bmu}{\boldsymbol{\mu}}\) \(\newcommand{\bSigma}{\boldsymbol{\Sigma}}\) \(\newcommand{\bfbeta}{\boldsymbol{\beta}}\) \(\newcommand{\bflambda}{\boldsymbol{\lambda}}\) \(\newcommand{\bgamma}{\boldsymbol{\gamma}}\) \(\newcommand{\bsigma}{{\boldsymbol{\sigma}}}\) \(\newcommand{\bpi}{\boldsymbol{\pi}}\) \(\newcommand{\btheta}{{\boldsymbol{\theta}}}\) \(\newcommand{\bphi}{\boldsymbol{\phi}}\) \(\newcommand{\balpha}{\boldsymbol{\alpha}}\) \(\newcommand{\blambda}{\boldsymbol{\lambda}}\) \(\renewcommand{\P}{\mathbb{P}}\) \(\newcommand{\E}{\mathbb{E}}\) \(\newcommand{\indep}{\perp\!\!\!\perp} \newcommand{\bx}{\mathbf{x}}\) \(\newcommand{\bp}{\mathbf{p}}\) \(\renewcommand{\bx}{\mathbf{x}}\) \(\newcommand{\bX}{\mathbf{X}}\) \(\newcommand{\by}{\mathbf{y}}\) \(\newcommand{\bY}{\mathbf{Y}}\) \(\newcommand{\bz}{\mathbf{z}}\) \(\newcommand{\bZ}{\mathbf{Z}}\) \(\newcommand{\bw}{\mathbf{w}}\) \(\newcommand{\bW}{\mathbf{W}}\) \(\newcommand{\bv}{\mathbf{v}}\) \(\newcommand{\bV}{\mathbf{V}}\) \(\newcommand{\bfg}{\mathbf{g}}\) \(\newcommand{\bfh}{\mathbf{h}}\) \(\newcommand{\horz}{\rule[.5ex]{2.5ex}{0.5pt}}\) \(\renewcommand{\S}{\mathcal{S}}\) \(\newcommand{\X}{\mathcal{X}}\) \(\newcommand{\var}{\mathrm{Var}}\) \(\newcommand{\pa}{\mathrm{pa}}\) \(\newcommand{\Z}{\mathcal{Z}}\) \(\newcommand{\bh}{\mathbf{h}}\) \(\newcommand{\bb}{\mathbf{b}}\) \(\newcommand{\bc}{\mathbf{c}}\) \(\newcommand{\cE}{\mathcal{E}}\) \(\newcommand{\cP}{\mathcal{P}}\) \(\newcommand{\bbeta}{\boldsymbol{\beta}}\) \(\newcommand{\bLambda}{\boldsymbol{\Lambda}}\) \(\newcommand{\cov}{\mathrm{Cov}}\) \(\newcommand{\bfk}{\mathbf{k}}\) \(\newcommand{\idx}[1]{}\) \(\newcommand{\xdi}{}\)
8.1. Motivating example: classifying natural images#
In this chapter, we return to the classification problem. This time we consider more complex datasets involving natural images. We have seen an example previously, the MNIST dataset. We use a related dataset known as Fashion-MNIST developed by the Zalando Research. Quoting from their GitHub repository:
Fashion-MNIST is a dataset of Zalando’s article images – consisting of a training set of 60,000 examples and a test set of 10,000 examples. Each example is a 28x28 grayscale image, associated with a label from 10 classes. We intend Fashion-MNIST to serve as a direct drop-in replacement for the original MNIST dataset for benchmarking machine learning algorithms. It shares the same image size and structure of training and testing splits.
Figure: Fashion-MNIST sample images (Source)
\(\bowtie\)
We first load the data and convert it to an appropriate matrix representation. The data can be accessed with torchvision.datasets.FashionMNIST
.
import torch
from torchvision import datasets, transforms
from torch.utils.data import DataLoader, TensorDataset
fashion_mnist = datasets.FashionMNIST(root='./data', train=True,
download=True, transform=transforms.ToTensor())
For example, the first image and its label are the following. The squeeze()
below removes the color dimension in the image, which is grayscale.
img, label = fashion_mnist[0]
plt.figure()
plt.imshow(img.squeeze(), cmap='gray')
plt.show()
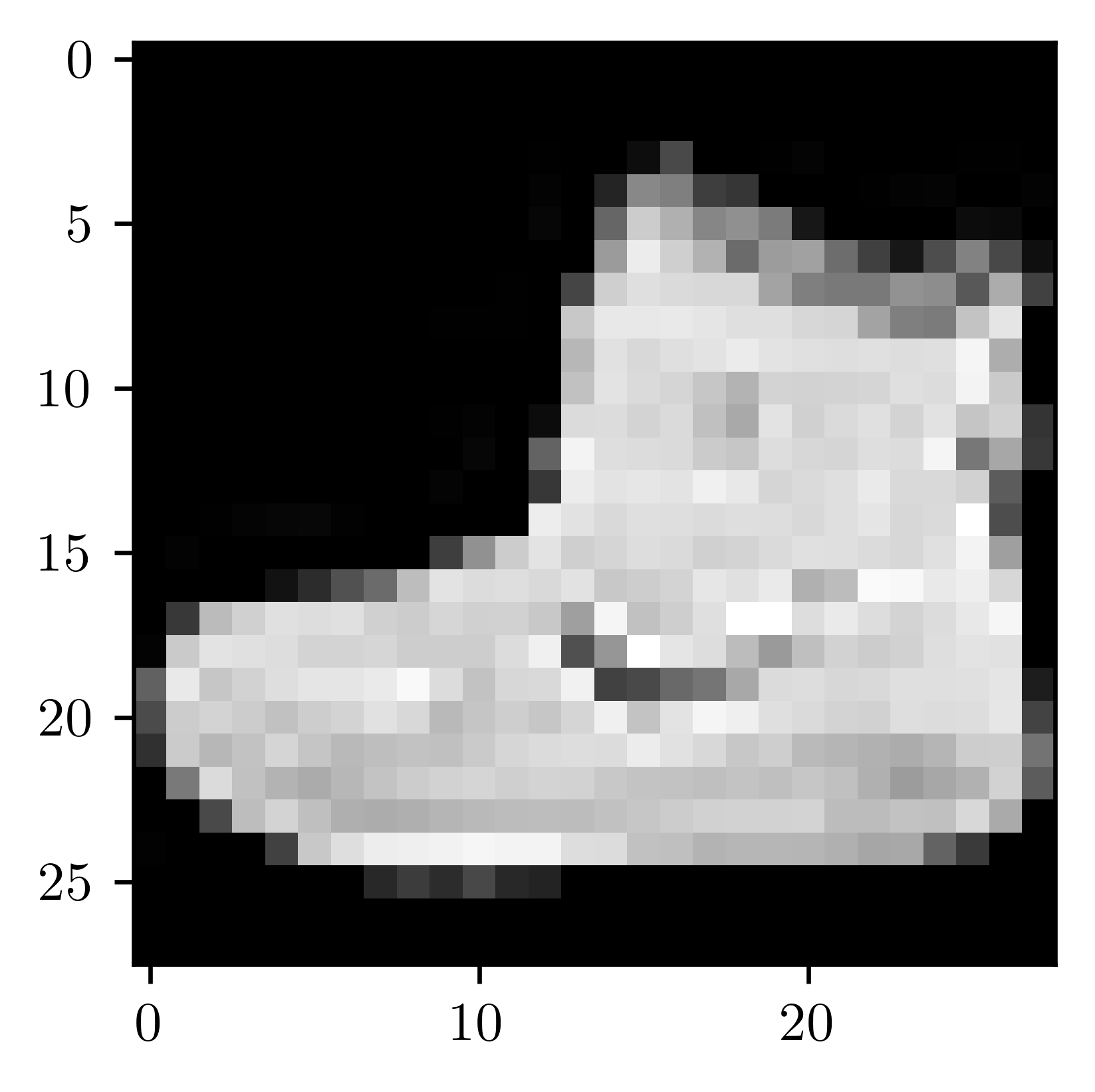
label
9
This label is not particularly meaningful. One can get the actual names of the classes as follows.
def FashionMNIST_get_class_name(label):
class_names = ["T-shirt/top", "Trouser", "Pullover", "Dress",
"Coat", "Sandal", "Shirt", "Sneaker", "Bag", "Ankle boot"]
return class_names[label]
print(f"{label}: '{FashionMNIST_get_class_name(label)}'")
9: 'Ankle boot'
The purpose of this chapter is to develop some of the mathematical tools used to solve this kind of classification problem:
neural networks,
backpropagation,
stochastic gradient descent.